热点文献带您关注AI计算机视觉——图书馆前沿文献专题推荐服务(13)
发布时间:2020-05-29
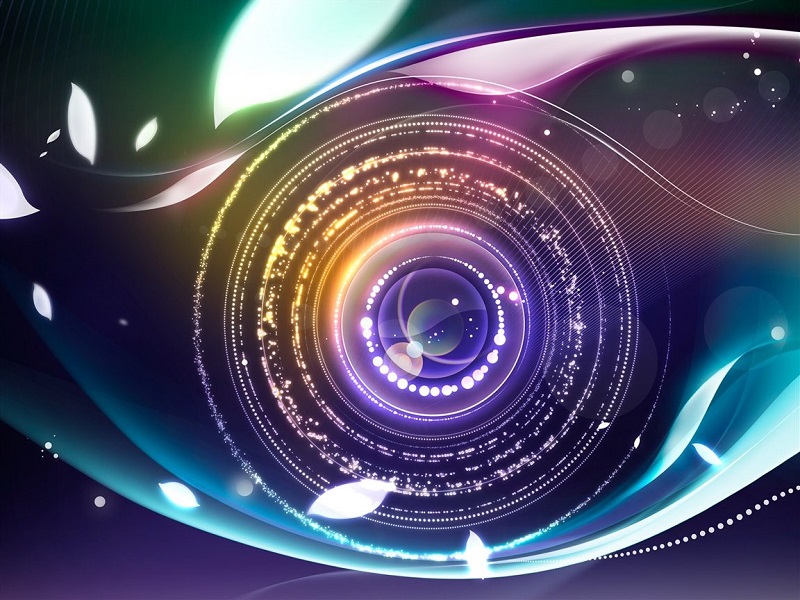
在上一期AI自然语言处理技术的推荐中,我们为您推荐了机器翻译的前沿论文。从本期推荐开始,我们将为您推荐计算机视觉领域的热点论文。
计算机视觉是人工智能的一个重要分支,在智能驾驶、安防、医疗等各行各业中得到了广泛的运用。如何再现人类视觉的能力,跟踪、识别、分析、处理并理解图像的内容,是计算机视觉的最终目标。
本期选取了4篇文献,介绍计算机视觉的最新动态,包括基于Grad-CAM的视觉解释、采用LPM消除图像特征中的不匹配对应、用于细胞图像分析的深度学习、使用深度学习识别遗传性疾病的面部表型等文献,推送给相关领域的科研人员。

Grad-CAM: Visual Explanations from Deep Networks via Gradient-Based Localization
Selvaraju, Ramprasaath R., etc.
INTERNATIONAL JOURNAL OF COMPUTER VISION, 2020, 128(2): 336-359
We propose a technique for producing 'visual explanations' for decisions from a large class of Convolutional Neural Network (CNN)-based models, making them more transparent and explainable. Our approach-Gradient-weighted Class Activation Mapping (Grad-CAM), uses the gradients of any target concept (say 'dog' in a classification network or a sequence of words in captioning network) flowing into the final convolutional layer to produce a coarse localization map highlighting the important regions in the image for predicting the concept. Unlike previous approaches, Grad-CAM is applicable to a wide variety of CNN model-families: (1) CNNs with fully-connected layers (e.g.VGG), (2) CNNs used for structured outputs (e.g.captioning), (3) CNNs used in tasks with multi-modal inputs (e.g.visual question answering) or reinforcement learning, all without architectural changes or re-training. We combine Grad-CAM with existing fine-grained visualizations to create a high-resolution class-discriminative visualization, Guided Grad-CAM, and apply it to image classification, image captioning, and visual question answering (VQA) models, including ResNet-based architectures. In the context of image classification models, our visualizations (a) lend insights into failure modes of these models (showing that seemingly unreasonable predictions have reasonable explanations), (b) outperform previous methods on the ILSVRC-15 weakly-supervised localization task, (c) are robust to adversarial perturbations, (d) are more faithful to the underlying model, and (e) help achieve model generalization by identifying dataset bias. For image captioning and VQA, our visualizations show that even non-attention based models learn to localize discriminative regions of input image. We devise a way to identify important neurons through Grad-CAM and combine it with neuron names (Bau et al. in Computer vision and pattern recognition, 2017) to provide textual explanations for model decisions. Finally, we design and conduct human studies to measure if Grad-CAM explanations help users establish appropriate trust in predictions from deep networks and show that Grad-CAM helps untrained users successfully discern a 'stronger' deep network from a 'weaker' one even when both make identical predictions.

Locality Preserving Matching
Ma, Jiayi, etc.
INTERNATIONAL JOURNAL OF COMPUTER VISION, 2019, 127(5): 512-531
Seeking reliable correspondences between two feature sets is a fundamental and important task in computer vision. This paper attempts to remove mismatches from given putative image feature correspondences. To achieve the goal, an efficient approach, termed as locality preserving matching (LPM), is designed, the principle of which is to maintain the local neighborhood structures of those potential true matches. We formulate the problem into a mathematical model, and derive a closed-form solution with linearithmic time and linear space complexities. Our method can accomplish the mismatch removal from thousands of putative correspondences in only a few milliseconds. To demonstrate the generality of our strategy for handling image matching problems, extensive experiments on various real image pairs for general feature matching, as well as for point set registration, visual homing and near-duplicate image retrieval are conducted. Compared with other state-of-the-art alternatives, our LPM achieves better or favorably competitive performance in accuracy while intensively cutting time cost by more than two orders of magnitude.

Deep learning for cellular image analysis
Moen, Erick, etc.
NATURE METHODS, 2019, 16(12): 1233-1246
Recent advances in computer vision and machine learning underpin a collection of algorithms with an impressive ability to decipher the content of images. These deep learning algorithms are being applied to biological images and are transforming the analysis and interpretation of imaging data. These advances are positioned to render difficult analyses routine and to enable researchers to carry out new, previously impossible experiments. Here we review the intersection between deep learning and cellular image analysis and provide an overview of both the mathematical mechanics and the programming frameworks of deep learning that are pertinent to life scientists. We survey the field's progress in four key applications: image classification, image segmentation, object tracking, and augmented microscopy. Last, we relay our labs' experience with three key aspects of implementing deep learning in the laboratory: annotating training data, selecting and training a range of neural network architectures, and deploying solutions. We also highlight existing datasets and implementations for each surveyed application.

Identifying facial phenotypes of genetic disorders using deep learning
Gurovich, Yaron, etc.
NATURE MEDICINE, 2019, 25(1): 60-+
Syndromic genetic conditions, in aggregate, affect 8% of the population(1). Many syndromes have recognizable facial features(2) that are highly informative to clinical geneticists(3-5). Recent studies show that facial analysis technologies measured up to the capabilities of expert clinicians in syndrome identification(6-9). However, these technologies identified only a few disease phenotypes, limiting their role in clinical settings, where hundreds of diagnoses must be considered. Here we present a facial image analysis framework, DeepGestalt, using computer vision and deep-learning algorithms, that quantifies similarities to hundreds of syndromes. DeepGestalt outperformed clinicians in three initial experiments, two with the goal of distinguishing subjects with a target syndrome from other syndromes, and one of separating different genetic sub-types in Noonan syndrome. On the final experiment reflecting a real clinical setting problem, DeepGestalt achieved 91% top-10 accuracy in identifying the correct syndrome on 502 different images. The model was trained on a dataset of over 17,000 images representing more than 200 syndromes, curated through a community-driven phenotyping platform. DeepGestalt potentially adds considerable value to phenotypic evaluations in clinical genetics, genetic testing, research and precision medicine.