热点文献带您关注AI计算机视觉 ——图书馆前沿文献专题推荐服务(15)
发布时间:2020-06-15
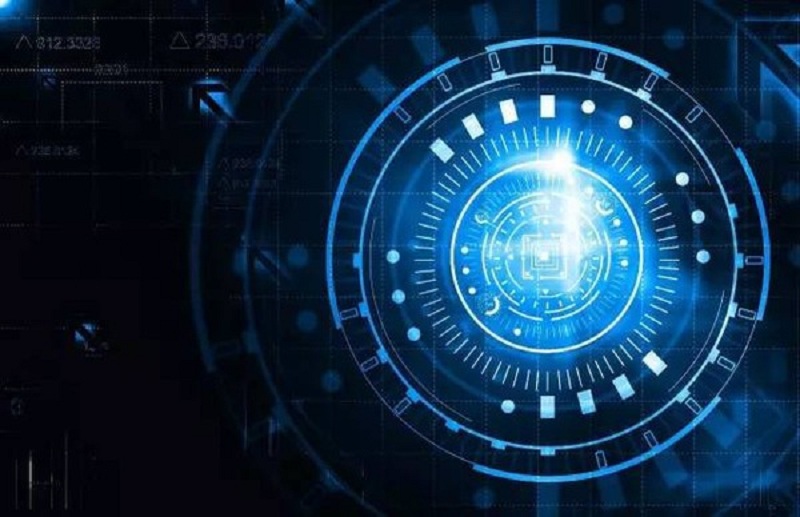
在上一期AI文献推荐中,我们为您推荐了计算机视觉领域的前沿论文。在本期推荐中,我们将继续为您推荐计算机视觉理解的热点论文。
细粒度图像分类是计算机视觉中一项基础的重要工作,通过图像前背景分离得到的细粒度图能够使计算机视觉系统完成更精准的预处理。此外,视频目标检测与识别、视觉场景的语义理解是计算机视觉的研究难点之一。将细粒度算法与视觉技术相结合,能够更好地解决视觉场景语义理解、视频目标跟踪、视频行为识别等技术领域的难题。
本期选取了4篇文献,介绍计算机视觉的最新动态,包括面向视觉理解的结构化标签、基于ADE20K数据集的视觉场景语义理解、无手眼标定的单参考图像ACR、深度集成网络在骨骼动作识别中的应用等文献,推送给相关领域的科研人员。

Structured Label Inference for Visual Understanding
Nauata, Nelson, etc.
IEEE TRANSACTIONS ON PATTERN ANALYSIS AND MACHINE INTELLIGENCE, 2020, 42(5): 1257-1271
Visual data such as images and videos contain a rich source of structured semantic labels as well as a wide range of interacting components. Visual content could be assigned with fine-grained labels describing major components, coarse-grained labels depicting high level abstractions, or a set of labels revealing attributes. Such categorization over different, interacting layers of labels evinces the potential for a graph-based encoding of label information. In this paper, we exploit this rich structure for performing graph-based inference in label space for a number of tasks: multi-label image and video classification and action detection in untrimmed videos. We consider the use of the Bidirectional Inference Neural Network (BINN) and Structured Inference Neural Network (SINN) for performing graph-based inference in label space and propose a Long Short-Term Memory (LSTM) based extension for exploiting activity progression on untrimmed videos. The methods were evaluated on (i) the Animal with Attributes (AwA), Scene Understanding (SUN) and NUS-WIDE datasets for multi-label image classification, (ii) the first two releases of the YouTube-8M large scale dataset for multi-label video classification, and (iii) the THUMOS'14 and MultiTHUMOS video datasets for action detection. Our results demonstrate the effectiveness of structured label inference in these challenging tasks, achieving significant improvements against baselines.
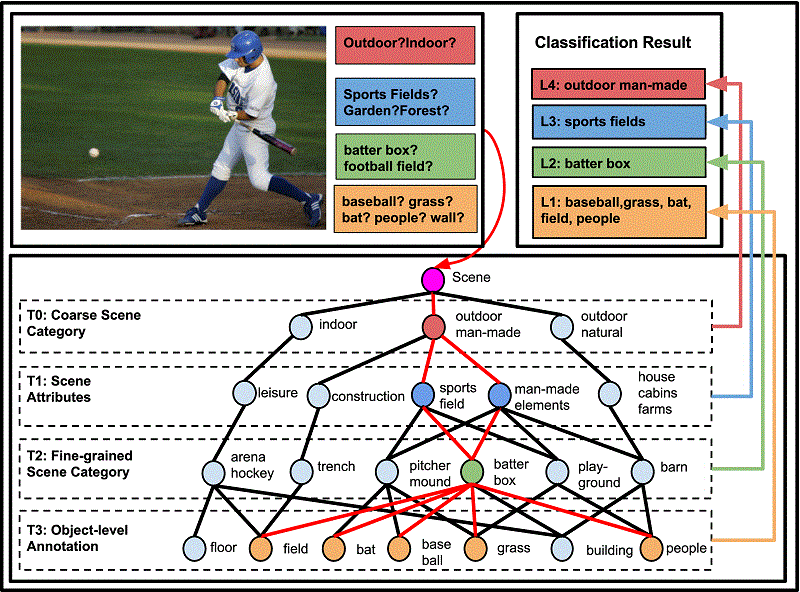

Semantic Understanding of Scenes Through the ADE20K Dataset
Zhou, Bolei, etc.
INTERNATIONAL JOURNAL OF COMPUTER VISION, 2019, 127(3): 302-321
Semantic understanding of visual scenes is one of the holy grails of computer vision. Despite efforts of the community in data collection, there are still few image datasets covering a wide range of scenes and object categories with pixel-wise annotations for scene understanding. In this work, we present a densely annotated dataset ADE20K, which spans diverse annotations of scenes, objects, parts of objects, and in some cases even parts of parts. Totally there are 25k images of the complex everyday scenes containing a variety of objects in their natural spatial context. On average there are 19.5 instances and 10.5 object classes per image. Based on ADE20K, we construct benchmarks for scene parsing and instance segmentation. We provide baseline performances on both of the benchmarks and re-implement state-of-the-art models for open source. We further evaluate the effect of synchronized batch normalization and find that a reasonably large batch size is crucial for the semantic segmentation performance. We show that the networks trained on ADE20K are able to segment a wide variety of scenes and objects.
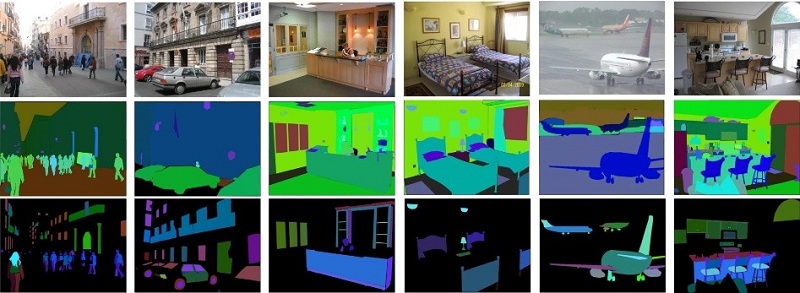

Active Camera Relocalization from a Single Reference Image without Hand-Eye Calibration
Tian, Fei-peng, etc.
IEEE TRANSACTIONS ON PATTERN ANALYSIS AND MACHINE INTELLIGENCE, 2019, 41(12): 2791-2806
This paper studies active relocalization of 6D camera pose from a single reference image, a new and challenging problem in computer vision and robotics. Straightforward active camera relocalization (ACR) is a tricky and expensive task that requires elaborate hand-eye calibration on precision robotic platforms. In this paper, we show that high-quality camera relocalization can be achieved in an active and much easier way. We propose a hand-eye calibration free approach to actively relocating the camera to the same 6D pose that produces the input reference image. We theoretically prove that, given bounded unknown hand-eye pose displacement, this approach is able to rapidly reduce both 3D relative rotational and translational pose between current camera and the reference one to an identical matrix and a zero vector, respectively. Based on these findings, we develop an effective ACR algorithm with fast convergence rate, reliable accuracy and robustness. Extensive experiments validate the effectiveness and feasibility of our approach on both laboratory tests and challenging real-world applications in fine-grained change monitoring of cultural heritages.

Deep ensemble network using distance maps and body part features for skeleton based action recognition
Naveenkumar, M., etc.
PATTERN RECOGNITION, 2020, 100
Human action recognition is a hot research topic in the field of computer vision. The availability of low cost depth sensors in the market made the extraction of reliable skeleton maps of human objects easier. This paper proposes three subnets, referred to as SNet, TNet, and BodyNet to capture diverse spatiotemporal dynamics for action recognition task. Specifically, SNet is used to capture pose dynamics from the distance maps in the spatial domain. The second subnet (TNet) captures the temporal dynamics along the sequence. The third net (BodyNet) extracts distinct features from the fine-grained body parts in the temporal domain. With the motivation of ensemble learning, a hybrid network, referred to as HNet, is modeled using two subnets (TNet and BodyNet) to capture robust temporal dynamics. Finally, SNet and HNet are fused as one ensemble network for action classification task. Our method achieves competitive results on three widely used datasets: UTD MHAD, UT Kinect and NTU RGB+D.