热点文献带您关注AI文本摘要自动生成——图书馆前沿文献专题推荐服务(9)
发布时间:2020-04-30
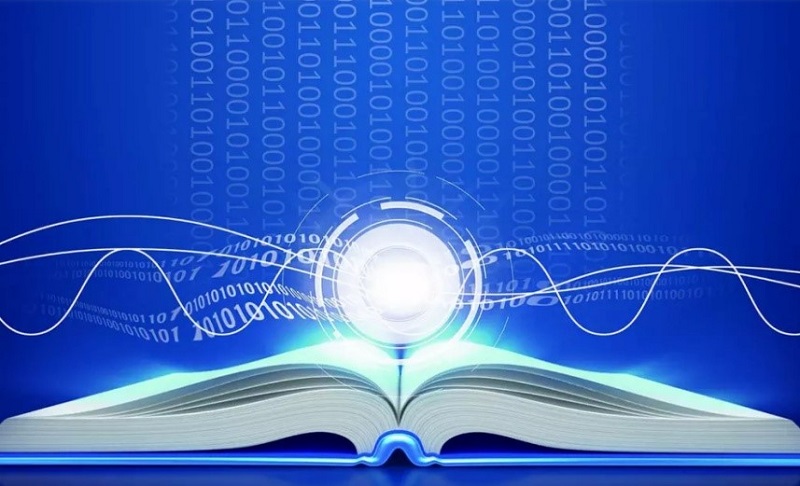
在上一期人工智能自然语言处理技术的推荐中,我们为您推荐了情感分类的技术与应用。在本期推荐中,我们将介绍自然语言处理的生成式任务之一——自动文本摘要生成。
自动文本摘要的生成方式通常可分为抽取式和生成式两类。抽取式文本摘要是从原文中抽取重要的句子形成摘要,生成式摘要则是在理解原文的基础上用新的语言生成摘要。随着深度学习的发展,自动生成摘要的实用性、准确性有了大幅提高。
本期选取了4篇文献,介绍自动文本摘要生成的最新动态,包括新型的句子嵌入框架,基于频繁项集和语义分析的文本摘要算法,基于sequence-to-sequence模型的双重编码,基于文本和多媒体数据的多模式摘要等,推送给相关领域的科研人员。

Jointly Learning Topics in Sentence Embedding for Document Summarization
Gao, Yang, etc.
IEEE TRANSACTIONS ON KNOWLEDGE AND DATA ENGINEERING, 2020, 32(4): 688-699
Summarization systems for various applications, such as opinion mining, online news services, and answering questions, have attracted increasing attention in recent years. These tasks are complicated, and a classic representation using bag-of-words does not adequately meet the comprehensive needs of applications that rely on sentence extraction. In this paper, we focus on representing sentences as continuous vectors as a basis for measuring relevance between user needs and candidate sentences in source documents. Embedding models based on distributed vector representations are oen used in the summarization community because, through cosine similarity, they simplify sentence relevance when comparing two sentences or a sentence/query and a document. However, the vector-based embedding models do not typically account for the salience of a sentence, and this is a very necessary part of document summarization. To incorporate sentence salience, we developed a model, called CCTSenEmb, that learns latent discriminative Gaussian topics in the embedding space and extended the new framework by seamlessly incorporating both topic and sentence embedding into one summarization system. To facilitate the semantic coherence between sentences in the framework of prediction-based tasks for sentence embedding, the CCTSenEmb further considers the associations between neighboring sentences. As a result, this novel sentence embedding framework combines sentence representations, word-based content, and topic assignments to predict the representation of the next sentence. A series of experiments with the DUC datasets validate CCTSenEmb's efficacy in document summarization in a query-focused extraction-based setting and an unsupervised ILP-based setting.

ELSA: A Multilingual Document Summarization Algorithm Based on Frequent Itemsets and Latent Semantic Analysis
Cagliero, Luca, etc.
ACM TRANSACTIONS ON INFORMATION SYSTEMS, 2019, 37(2)
Sentence-based summarization aims at extracting concise summaries of collections of textual documents. Summaries consist of a worthwhile subset of document sentences. The most effective multilingual strategies rely on Latent Semantic Analysis (LSA) and on frequent itemset mining, respectively. LSAbased summarizers pick the document sentences that cover the most important concepts. Concepts are modeled as combinations of single-document terms and are derived from a term-by-sentence matrix by exploiting Singular Value Decomposition (SVD). Itemset-based summarizers pick the sentences that contain the largest number of frequent itemsets, which represent combinations of frequently co-occurring terms. The main drawbacks of existing approaches are (i) the inability of LSA to consider the correlation between combinations of multiple-document terms and the underlying concepts, (ii) the inherent redundancy of frequent itemsets because similar itemsets may be related to the same concept, and (iii) the inability of itemset-based summarizers to correlate itemsets with the underlying document concepts. To overcome the issues of both of the abovementioned algorithms, we propose a new summarization approach that exploits frequent itemsets to describe all of the latent concepts covered by the documents under analysis and LSA to reduce the potentially redundant set of itemsets to a compact set of =correlated concepts. The summarizer selects the sentences that cover the latent concepts with minimal redundancy. We tested the summarization algorithm on both multilingual and English-language benchmark document collections. The proposed approach performed significantly better than both itemset- and LSA-based summarizers, and better than most of the other state-of-the-art approaches.

Dual Encoding for Abstractive Text Summarization
Yao, Kaichun, etc.
IEEE TRANSACTIONS ON CYBERNETICS, 2019, 50(3): 985-996
Recurrent neural network-based sequence-to-sequence attentional models have proven effective in abstractive text summarization. In this paper, we model abstractive text summarization using a dual encoding model. Different from the previous works only using a single encoder, the proposed method employs a dual encoder including the primary and the secondary encoders. Specifically, the primary encoder conducts coarse encoding in a regular way, while the secondary encoder models the importance of words and generates more fine encoding based on the input raw text and the previously generated output text summarization. The two level encodings are combined and fed into the decoder to generate more diverse summary that can decrease repetition phenomenon for long sequence generation. The experimental results on two challenging datasets (i.e., CNN/DailyMail and DUC 2004) demonstrate that our dual encoding model performs against existing methods.

Read, Watch, Listen, and Summarize: Multi-Modal Summarization for Asynchronous Text, Image, Audio and Video
Li, Haoran, etc.
IEEE TRANSACTIONS ON KNOWLEDGE AND DATA ENGINEERING, 2019, 31(5):996-1009
Automatic text summarization is a fundamental natural language processing (NLP) application that aims to condense a source text into a shorter version. The rapid increase in multimedia data transmission over the Internet necessitates multi-modal summarization (MMS) from asynchronous collections of text, image, audio, and video. In this work, we propose an extractive MMS method that unites the techniques of NLP, speech processing, and computer vision to explore the rich information contained in multi-modal data and to improve the quality of multimedia news summarization. The key idea is to bridge the semantic gaps between multi-modal content. Audio and visual are main modalities in the video. For audio information, we design an approach to selectively use its transcription and to infer the salience of the transcription with audio signals. For visual information, we learn the joint representations of text and images using a neural network. Then, we capture the coverage of the generated summary for important visual information through text-image matching or multi-modal topic modeling. Finally, all the multi-modal aspects are considered to generate a textual summary by maximizing the salience, nonredundancy, readability, and coverage through the budgeted optimization of submodular functions. We further introduce a publicly available MMS corpus in English and Chinese. 1 The experimental results obtained on our dataset demonstrate that our methods based on image matching and image topic framework outperform other competitive baseline methods.